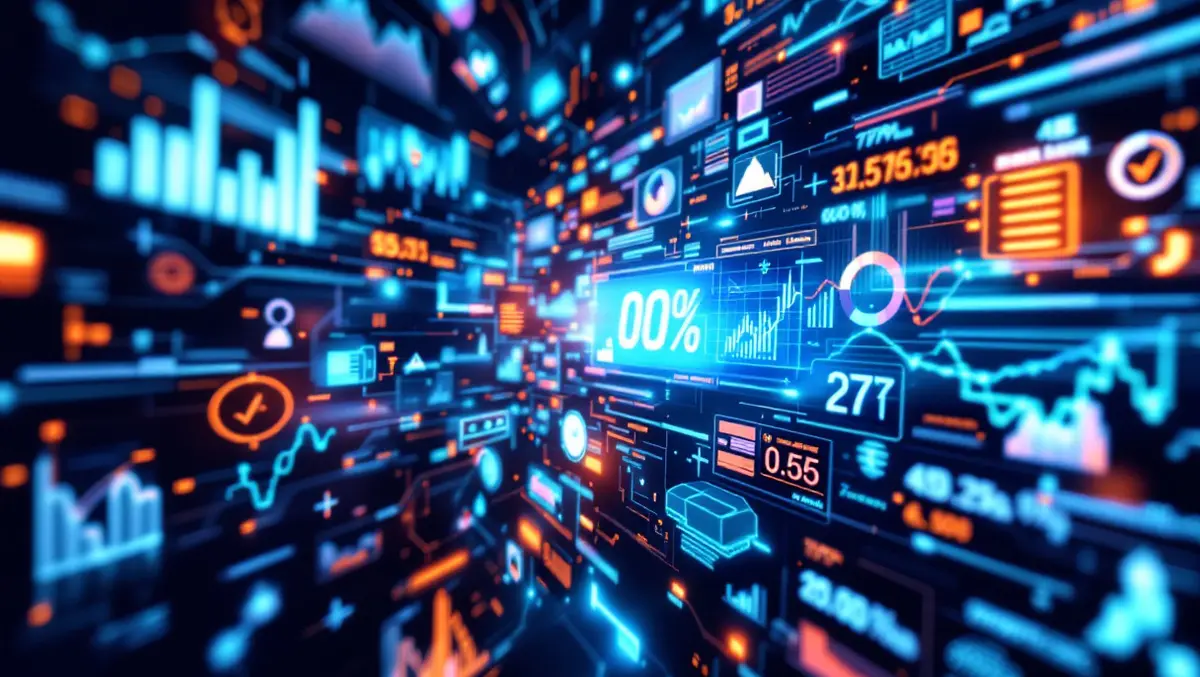
Managing and optimising soaring levels of smart label data
As the adoption of smart labels starts to take hold, enterprises are beginning to amass a huge volume of data. Once collated and analysed, this data has the potential to bring value to the company in question, providing prized insights that can help radically improve operational performance. But how do enterprises manage and process the data gathered? And how is the industry evolving with regard to smart label data sharing?
Surge in smart label data
Slim, energy-efficient smart labels have only been around for a few years in earnest; the first smart labels were only introduced in 2023. Yet despite only being in their infancy, adoption of smart labels is already beginning to take hold, with analysts predicting that the technology will surge in the coming years. According to Precedence Research, the global smart label market grew from $14.10 billion in 2024 to $16.41 billion in 2025 and is projected to reach around USD 64.42 billion by 2034. And with this surge in adoption of smart labels comes an almighty surge in smart label-generated data. If we consider each label to generate approximately 0.5-1MB of data on average during its lifespan, tens of millions of labels would collectively produce dozens of terabytes of data per year.
In order to derive value from this potential gold mine of smart label data, business intelligence and analysis platforms are fundamental. Indeed, the two go hand-in-hand; without a smart means of collating and assessing the data from smart labels, the use of these devices is practically meaningless. Smart labels collate detailed data logs, which provide fodder for business intelligence tools. Once analysed, management teams can use this data to make informed decisions to improve their business's operations and performance.
To take a real-life example, a mobile phone retailer can use smart labels on imports of iPhones to its central warehouse to better manage the distribution of phones to retail outlets across the country. If, for example, it can see that the stock of black iPhone 16s is low at one particular retail outlet, it can predict that over the next few days the store will run out of devices and take action to ensure that the phones are distributed from the warehouse according to stock levels and anticipated sales. The ability to take smart label data into wider consideration will drive improved business performance.
As smart label data becomes regularly analysed by BI systems, the next stage will be to use AI to take appropriate and automated actions, with AI decision-making improving with every data set analysed by the BI/AI system. Indeed, AI will become indispensable in methodically managing and taking actions based on the surging volume of smart label data as device implementation soars.
Data sharing across the ecosystem
We are still at the initial stages of smart label adoption, with organisations implementing their own solutions for their specific business or operational needs. This means that within a supply chain scenario, there could be numerous smart labels on dispatched items, each reporting data to an individual player (such as a road haulage firm) responsible for the goods for a particular part of their transit.
As smart label technologies and platforms mature, this siloed data-gathering approach will have limitations. Industries would gain more from implementing these technologies if data assets could be shared among the ecosystem. In the case of the iPhone retailer, this would mean having a single smart label gathering a 'single source' of data that could be used by all stakeholders (including, in this instance, transport or courier companies) so that they could also gather insights relevant to their specific competence or responsibility. It would eliminate inconsistencies in data gathering, provide a more sustainable solution, and help drive innovation by bringing diverse players together.
Of course, data sharing across the ecosystem has its own set of challenges. Stakeholders would need to set up a pool of resources to manage the infrastructure and devices, data security would need to be watertight, and access to this data would need to be well-controlled and monitored. Aside from the technical issues, there are cultural challenges to sharing data with other unknown parties and 'letting go' of control that must be overcome.
Today, however, enterprises considering implementing smart labels must focus on ensuring that the full value of the generated data is optimised and acted upon. Once they have begun to reap the benefits of evaluating the data using business intelligence to improve their performance actively, they can explore the benefits of using AI to automate and streamline decision-making based on these smart label data insights. Beyond this, they should look at ways to work alongside the ecosystem to share smart label data for a more sustainable, collaborative future. While this may seem like blue-sky thinking, the evolution of smart labels is moving so quickly that this 'futuristic approach' to collaborative use of generated data is much closer than we think.